1. Introduction
This article discusses the management issues involved in introducing generative AI technology to organizations, such as companies, from the perspective of knowledge creation, given the current circumstances under which the widespread use of generative AI technology is becoming feasible. In particular, I present a discussion on the fact that developing the technological content that is then standardized is a knowledge creation process, which is difficult to perform using generative AI technology. As a source of knowledge for this creation process, attention is directed toward the information that is obtainable through participation in standardization activities of a standards development organization (SDO). In this context, standardization refers to the technical agreements formed between participants in an SDO (Note 1, Note 2).
The explanation presented here utilizes the results of a questionnaire survey (Survey on Standardization Activities [SoSA) targeting companies in Japan (Appendix Tables A.1. and A.2.). Prior to the 2021 survey, the SoSA was administered four times, covering the period from 2017 to 2020 (Tamura, 2019, 2020, 2021, 2022a; Note 3, Note 4, Note 5, Note 6).
2. Background
Human history can be regarded as a history of knowledge creation, encompassing the development of new knowledge in both the natural sciences and the humanities. The efficient creation and management of innovative knowledge have long been a central topic of interest in both academia and practice, long before the advent of generative AI technology (Nonaka and Takeuchi, 1995; Stewart, 1999).
Two types of knowledge are commonly recognized: explicit knowledge (formal knowledge that has been documented in text) and implicit (tacit) knowledge that remains undocumented (Polanyi, 1966). In the context of innovation creation, there is significant interest in clarifying the impact of documented knowledge sources—such as patents, academic papers, and academic books—on the generation of new knowledge, as these are often viewed as reservoirs of scientific and technological information (Ochi, Shiro, Mori, and Sakata, 2022). The increasing volume of text data available on the Internet, combined with advances in Natural Language Processing (NLP), enables the measurement of citation relationships between documents and the assessment of similarities between them. Through analyzing these relationships, meta-analyses of the flow of knowledge among authors and the knowledge structure within specific domains have become feasible (Tamura, Iwami, and Sakata, 2021; Appendix Table B.1.). However, such analysis requires that the analyzed knowledge be available as text data (i.e., formal knowledge).
3. Important Sources of Knowledge in Standardization Activities
It is useful to determine which knowledge sources are regarded as important for standardization. Previous results compare the perceived importance of text-based information sources—such as “patent information,” “academic articles,” and “standardization documents”—with non-text-based sources, such as “information obtained from participation in standardization activities” (Table 1; Tamura, 2023).
Non-text-based knowledge related to SDO activities can be considered implicit knowledge, and approximately 62% of SoSA respondents consider it useful. On the other hand, knowledge obtained from standardized documents, which represents explicit knowledge, is considered useful by about 57% of SoSA respondents. This result suggests that both explicit knowledge (e.g., from standardization documents) and implicit knowledge (e.g., non-text information shared during standards’ development activities) play significant roles in standardization. These findings are likely related to the development of de jure standards and consortium standards, respectively, as processes that necessitate consensus-building among participants.
Generative AI employs large-scale language models (LLMs) to statistically estimate the appearance probability of each word in word sequences and produce documents as output (Brown et al., 2020). Non-verbal information from SDO meeting participants, which may include signals related to decision-making, cannot be readily captured as data. Even if some portion of this information is recorded in meeting minutes, it is likely to be limited and potentially introduces interpretive noise. As a result, such AI technology cannot generate knowledge that relies on processing non-verbal information. Even if the amount of implicit information becomes smaller in the future, such information will continue to exist. In other words, compared to explicit knowledge-intensive creation, tacit knowledge-intensive creation poses greater challenges for generative AI technology.
Executives generally do not carry out front-line work themselves and are therefore not able to gather information on the content of the work to the same extent as the employees who carry out this work. This situation creates a risk of information asymmetry between management and staff. Accordingly, the reallocation of managerial resources within an organization along with the introduction of generative AI technology must take into account the importance of tacit knowledge (for example, the standard development process described here). Furthermore, the process must be carried out with a clear understanding of which job responsibilities cannot be replaced by generative AI technology. From a management perspective, identifying “worker responsibilities that cannot be replaced” by generative AI technology is more crucial than identifying “tasks that can be replaced.”
The above considerations have substantial implications for Japan. In many Japanese industries, the practice of creating job descriptions is very limited, and the work content often remains undocumented. Under such conditions, accurately determining whether an individual’s work content can be performed by AI technology—including generative AI—is difficult. In other words, the information asymmetry persists, increasing the risk of poor decision-making regarding internal human resource allocation. This situation potentially leads to reduced productivity.
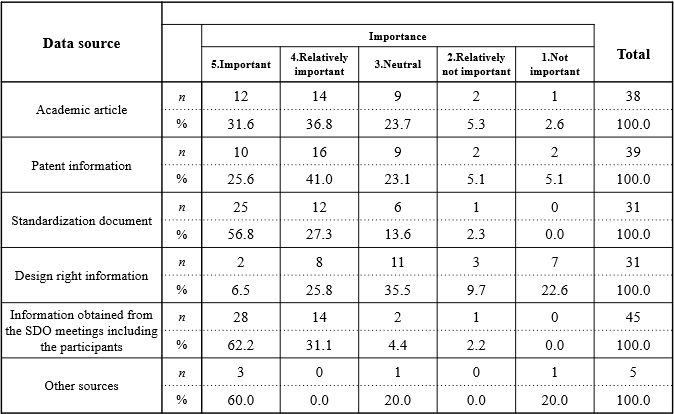
4. Conclusion
This discussion has examined the effects and limitations of generative AI technology on knowledge creation, using standard development as an example of knowledge creation that may be difficult for generative AI to handle. While AI technology can be utilized to enhance competitiveness if applied correctly, inappropriate implementation can lead to superficial formalism in the use of generative AI. When applying NLP-based information processing technologies and theories (e.g., LLM-based generative AI), it is essential to recognize that generative AI tools are not suited to certain knowledge creation processes. In particular, for organizations whose business strategies depend heavily on the standardization of goods and services, the knowledge creation work inherent in standardization activities (standard development) cannot be fully replaced by generative AI technology. In such cases, cultivating and enhancing human capital is especially critical.
Many Japanese companies do not have well-developed job description systems in place. The widespread adoption of generative AI technology may serve as an external shock that encourages changes in current human capital management systems, potentially accelerating a shift toward more direct managing of employee capabilities. From a business perspective, creating job descriptions for each position is important. As a management technique, improving conventional job description formats by including a checkbox to indicate whether generative AI implementation is “suitable” or “not suitable” for given tasks.
Implementing these measures appropriately requires that personnel responsible for human capital management possess a certain level of technical understanding of generative AI technology. In other words, a discontinuous change in the knowledge required of those who plan human capital management must occur. At a minimum, familiarity with NLP techniques in use among AI technologies will be essential for managers who are in charge of human capital allocation.
Appendix A
The distribution of survey respondents by industry classification and R&D budget is presented in Appendix Tables A.1. and A.2. A relatively large number of respondents belong to the manufacturing sector (e.g., steel, chemicals), the electrical machinery sector, and the non-manufacturing sector (e.g., transportation). Respondents selected their industry classification from ten different categories. This classification differs from the technical classifications utilized in JIS and ISO standards, which rely on technical differences rather than industry-based distinctions.
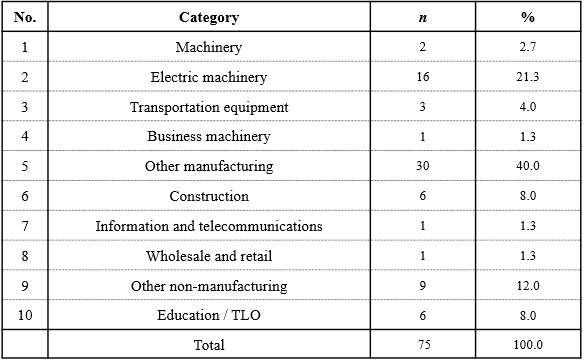
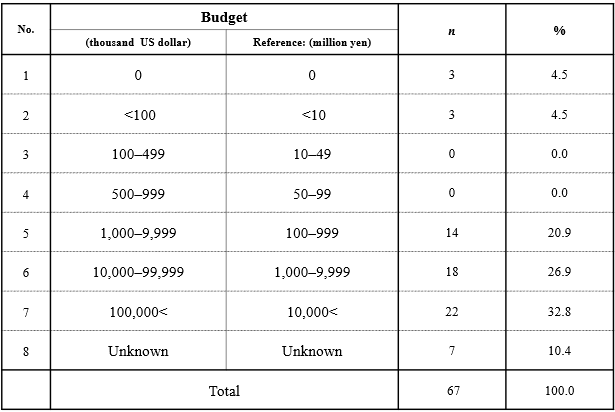
Note 2: Due to rounding, the simple sum of the percentages may not equal 100%.
Appendix B
![Figure B.1. Example of a meta-analysis using NLP (Cluster analysis of patents related to image technology [MPEG] standards) Figure B.1. Example of a meta-analysis using NLP (Cluster analysis of patents related to image technology [MPEG] standards)](/en/columns/data/a01_0774_figure_b1.png)