Lately, people have begun to point to the importance of evidence-based policymaking. The idea is that evidence-based discussion, supported by scientifically rigorous data analysis, should inform governments in their decisions in formulating and changing policies. The concept of evidence-based policy (EBP), initiated in the United States and the United Kingdom, has spread to other countries. In recent years, Japanese government ministries and agencies have been increasingly showing interest.
In order to accelerate the move toward evidence-based policymaking, government policymakers, as the users of evidence, need to have the ability to understand data analysis results accurately as well as detecting false results so as not to be deceived. However, a recent survey has found that such understanding is far from being widespread.
In this article, I would like to consider what is the "power of data analysis" that is essential to evidence-based policymaking, partly drawing on my recent book (Ito 2017).
Lack of ability to understand data analysis posing an obstacle to evidence-based policymaking
RIETI conducted a set of surveys to find out the actual state of evidence-based policymaking in Japan (Morikawa, 2017). One particularly interesting finding is how policymakers and policy researchers responded to the question asking what factors they think are posing an obstacle to evidence-based policymaking.
Approximately two-thirds of the respondents in both groups cited "Government officials are not skilled enough to analyze statistical data and understand relevant research findings," pointing to the need to enhance the analytical skills of government officials as essential infrastructure for evidence-based policymaking.
Importance of distinguishing causality from correlation
Although various skills are required to analyze statistical data and understand the resulting research findings, particularly important is the ability to determine whether a relationship identified by data analysis is a mere correlation or causality between policy interventions and outcomes.
Let's consider a specific example. Suppose that your boss asked you to find evidence relevant to the policy question of whether subsidies for energy conservation measures are effective in encouraging businesses to conserve energy. Thus, you gather relevant historical data.
By examining the data, you find the following. In 2011, Company A applied for and received a five million yen subsidy for energy conservation. Data aggregated for Company A show that its energy consumption per unit of production decreased from 100 in 2010 to 80 in 2012.
Based on this evidence, you make the following report to your boss:
"As shown in the figure below, Company A's energy consumption per unit of production decreased by 20% because of the effects of the subsidy provision. Therefore, the provision of energy conservation subsidies is expected to have significant energy-saving effects."
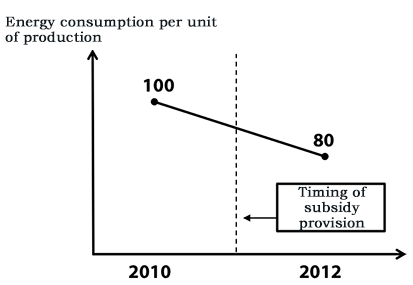
Now, let's consider why this conclusion may be false. You want to show the effects of energy conservation subsidies on energy consumption based on the results of data analysis. However, apart from the provision of subsidies, various other factors may have affected energy consumption in 2010 and 2012.
For instance, energy consumption for air conditioning at factories might have decreased because of a cooler summer in 2012 than in 2010. Employees of Company A might have become more conscious of the need to conserve energy in the wake of the Great East Japan Earthquake in 2011. Or, perhaps Company A introduced new, energy-efficient equipment in 2011 as had been planned regardless of the availability of the subsidy. Thus, from this data analysis, we cannot conclude that the provision of the subsidy caused Company A to improve its energy efficiency by 20%.
In other words, while the above figure shows a correlation between the provision of energy conservation subsidies and energy consumption, we cannot tell whether there is causality between the two factors. We can exclude the influence of some factors, such as differences in weather conditions, for which data are available. However, data on changes in employees' awareness of the need for energy conservation and those pointing to the fact that a certain company was to make capital investment in 2011 regardless are usually not available. Unless we exclude the influence of these factors, we cannot say that the provision of subsidies is effective as a measure to promote energy conservation by citing the correlation.
Why do we need to distinguish causality from correlation?
Mistaking mere correlation for causality in policymaking could result in a waste of taxes by deriving the conclusion that an ineffective policy measure has been effective. Such misinterpretation could also work in the opposite direction with a highly effective policy measure pronounced to have been ineffective, leading to a conclusion that calls for the abolishment of the policy measure.
When thus explained, it is relatively easy to understand that we should not take the presence of correlation as evidence in policymaking. In reality, however, the results of data analysis provided by those referred to as "specialists in data analysis" at the forefront of policymaking are mostly cases of mere correlation, and many of them are presented as "evidence" showing causality. This makes it all the more important for policymakers as users of such evidence to understand the difference between mere correlation and causality.
What sorts of data analysis can identify the causal effects of policy interventions?
The U.S. Commission on Evidence-Based Policymaking, launched under the Barack Obama administration, has been promoting the utilization of a data analysis approach that can provide scientific evidence of causality between policy interventions and outcomes.
The "randomized controlled trial (RCT)" method provides the most reliable scientific testing. One case example of the use of RCTs in Japan is the Agency for the Natural Resources and Energy's Next-Generation Energy and Social Systems Demonstration Project in which I was involved. Meanwhile, the "natural experiment" method, which takes advantage of a real world setting similar to an unnatural setting prepared specifically for a social experiment and can be implemented even in cases where RCTs are unfeasible, has continued to evolve.
Those readers interested in natural experiments may refer to Ito (2017) for more details. Here, I would like to draw attention to an important international trend. That is, U.S. and UK policymakers practicing evidence-based policymaking are increasingly demanding that evidence providers conduct scientific analysis to support evidence. In extreme cases, policymakers (such as the U.S. Department of Energy) have gone so far as to issue an order that no analytical findings be admitted as evidence unless supported by scientific analysis. By imposing stringent demands on those providing analysis results, policymakers are creating trends toward policy discussion based on highly reliable evidence.
Attempts to utilize evidence derived from scientific research in actual policymaking have been made in various countries. For instance, the California Public Utilities Commission (CPUC) used research findings from a researcher at the University of California, Berkeley as well as mine in its policymaking meeting (Borenstein, 2012; Ito, 2014). At the meeting, the commission pointed to the need to simplify and clarify the electricity rate structure by citing the analytical findings showing that a complex rate structure would cause confusion on the part of consumers and fail to deliver the intended policy effects, and this led to the simplification and clarification of electricity rate structure in California (CPUC, 2015). In another similar example, the Inter-American Development Bank (IDB) terminated its One Laptop per Child program and moved on to try out new development assistance programs after scientific analysis showed that the provision of free laptops did not have its intended effects on children in developing countries (Cristia et al., 2012). Meanwhile, the University of Chicago Crime Lab and the city of Chicago have been making continuous efforts to jointly analyze the effects of various policy interventions designed to prevent youths from developing criminal behavior in a bid to promote cost-effective measures by having cost-ineffective ones curtailed or eliminated (Heller et al., 2015).
Following these examples, Japan should work to produce reliable evidence and start discussing how we can make effective use of such evidence in actual policymaking.