Author Name | Hazem KRICHENE (University of Hyogo) / ARATA Yoshiyuki (Fellow, RIETI) / Abhijit CHAKRABORTY (University of Hyogo) / FUJIWARA Yoshi (University of Hyogo) / INOUE Hiroyasu (University of Hyogo) |
---|---|
Research Project | Large-scale Simulation and Analysis of Economic Network for Macro Prudential Policy |
Download / Links |
This Non Technical Summary does not constitute part of the above-captioned Discussion Paper but has been prepared for the purpose of providing a bold outline of the paper, based on findings from the analysis for the paper and focusing primarily on their implications for policy. For details of the analysis, read the captioned Discussion Paper. Views expressed in this Non Technical Summary are solely those of the individual author(s), and do not necessarily represent the views of the Research Institute of Economy, Trade and Industry (RIETI).
Industry Frontiers (FY2016-FY2019)
Large-scale Simulation and Analysis of Economic Network for Macro Prudential Policy
The recent economic and financial crisis have shown the high complexity of economic systems as reflected by the bounded rationality of agents (firms, households, government, etc.). This economic complexity was represented in several works as a network system which models the microscopic interaction between economic agents. Consequently, the economic fluctuations are the outcomes of suppliers-customers' interactions as discussed in Gabaix [2011]. For the Japanese economy, the production network constitutes the backbone of the trading interactions between suppliers and customers. How are these interactions formed between economic partners?
These interactions or the selection of economic partners may depend on different attributes such as geographic location, profit, financial health, etc. However, in case of production networks, it depends also on social links (relationships which already exist). In fact, a firm may select a partner based on a mutual relationship or existence of common partners. This reality makes the system evolve under endogeneity (see Jackson et al. [2017]).
In the case of an absence of endogeneity, a logistic regression is sufficient to explain how links are formed between firms. However, under endogeneity, we apply a regression based on the Exponential Random Graph Model (ERGM) proposed by Snijders [2002]. The ERGM technique requires very large simulations which constitute a constraint in estimating a large-scale network as the production network. To our knowledge, only small economic networks have been estimated (average dimension 100, see Lomi and Pattison [2006], Lomi and Fonti [2012]).
To overcome this limit, we developed a "fast" ERGM inspired by recent development in the literature and considered a significant sample of firms of the production network. In fact, we considered the Tokyo Stock Exchange (TSE) production network presenting the supplier-customer relationships between listed firms. The TSE production network has 3,198 firms and 20,417 links, and represents 22% of the total sales of the whole production network.
In the ERGM estimation, we consider 14 attributes divided in two sets: social attributes (endogenous) and economic attributes (exogenous). Social attributes are presented by degree (number of links), reciprocity (number of mutual links), in-stars (popularity: number of internal links), out-stars (activity: number of external links), transitivity (number of common partners), and two-path (number of external and internal links for each firm). Economic attributes are presented by sector homophily (number of links between firms with same sector of activity), geographic location homophily (number of links between firms with same geographic location), profit heterophily (difference of profit between connected firms), profit sender effect (activity depending on profit), and profit receiver effect (popularity depending on profit).
Estimation results are given in Table 1. All social parameters reflect a significant presence of social attachment. The likelihood of links formation increases by the reciprocity, transitivity, and activity and popularity of firms.
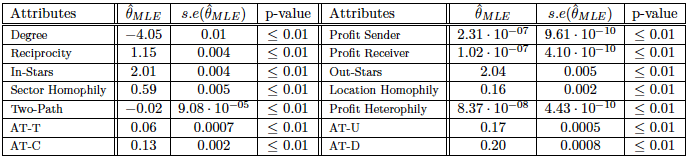
In fact, the positive reciprocity, triangles, and stars indicate that firms are more likely to establish links in the case of mutual trading, common partners, or popular and active partners. Moreover, all exogenous attributes are significant. In terms of sector, suppliers and customers from the same sector are more likely to exchange goods and services between them. This may make trading easier, i.e., firms with the same economic activity have similar interests. However, the economy presents systemic sector-based risk. If a disaster happens in one firm of sector A, a high contagion effect could be observed among other firms of that sector. In addition, firms choose partners which belong to the same geographic location (prefecture). This choice can be explained by the lower transportation cost. However, the economy presents a significant systemic geographic-based vulnerability. In case of Japan, this vulnerability could be dramatic especially with the high risk of natural disasters which can induce firms in the same prefecture to a cascading avalanche of bankruptcies. Finally, firms with higher profits are more likely to be active and popular (profit sender and receiver effects). The positive profit heterophily indicates that firms with low profits tend to be connected to those with high profits. This mechanism stimulates the emergence of hubs in the production network.
ERGM is a powerful model that estimates the TSE production network under the presence of endogeneity. It allows the quantification of the likelihood of edge formation based on several attributes. However, this model gives only statistical explanation and lacks economic interpretation such as the impact of the variation of parameters on the real economy (GDP, inflation, etc.).
- Reference(s)
-
- X. Gabaix. "The Granular Origins of Aggregate Fluctuations." Econometrica, 79(3):733–772, 2011.
- M.O. Jackson, B.W. Rogers, and Y. Zenou. "The Economic Consequence of Social-Network Structure." Journal of Economic Literature, 55(1):49–95, 2017.
- A. Lomi and F. Fonti. "Networks in Markets and the Propensity of Companies to Collaborate: An empirical test of three mechanisms." Economics Letters, 114:216–220, 2012.
- A. Lomi and P. Pattison. "Manufacturing Relations: An empirical study of the organization of production across multiple networks." Organization Science, 17(3):313–332, 2006.
- T.A.B. Snijders. "Markov Chain Monte Carlo Estimation of Exponential Random Graph Models." Journal of Social Structure, 3:1–40, 2002.