We do not know which characteristics affect cryptocurrency prices, if any. The column argues that there are two fundamental factors that drive prices in the long run: the trustworthiness of the cryptocurrency's blockchain and the adoption of the blockchain. Cryptocurrencies such as Bitcoin, Ethereum, and Monero are affected by these fundamentals. In some periods prices deviate, but eventually retrace the trend.
Identifying the determinants of asset prices is one of the most important questions in finance. Traditional asset pricing theories are founded on the idea that equity prices should be determined by fundamentals such as earnings (Gordon 1959, Campbell and Shiller 1988). In contrast, the behavioral finance literature posits that prices do not always follow fundamentals due to investor sentiment trading (Shiller 1981, Baker and Wurgler 2006, Stambaugh et al. 2012). Eventually however, equity prices seem to trace their fundamentals (Pa´stor and Veronesi, 2003, 2006, Bartram and Grinblatt 2018).
The price of cryptocurrency
In the case of cryptocurrencies, academia has barely scratched the surface with respect to identifying the determinants of their prices. For example, studies by Cheah and Fry (2015) and Corbet et al. (2018) claim that Bitcoin has no intrinsic value and that its price has persistently exhibited ‘bubble-like’ behaviour. Makarov and Schoar (2018) find that the prices of Bitcoin, Ethereum, and Ripple differ across exchanges for weeks. Outside of academia, the President of the United States recently tweeted that cryptocurrencies are based on "thin air" (Note 1).
In Bhambhwani et al. (2019), we challenge the perception that cryptocurrency markets are simply plagued with bubbles and speculative trading by identifying two key blockchain measures that affect cryptocurrency prices. Specifically, theory suggests that the trustworthiness and the transaction benefits of a blockchain are important determinants of cryptocurrency values. Pagnotta and Buraschi (2018) link trustworthiness to the computing power devoted to the blockchain. Biais et al. (2018) link transaction benefits of a cryptocurrency to the size of its network.
The importance of computing power and network for cryptocurrency prices is intuitive. From the supply side, computing power is provided by geographically dispersed miners that power the blockchain and are incentivised to do so as they are rewarded in units of the cryptocurrency that they ‘mine’. High levels of computing power allow transactions to flow efficiently, secure the blockchain from rogue attackers, and thus increase the trustworthiness of the blockchain. From the demand side, consumers value a blockchain as it provides censorship-resistant, border-less, and secure transactions. A larger network of users implies greater acceptability of the cryptocurrency as a medium of exchange as well as higher liquidity. Overall, computing power and network should be positively related to prices.
Investigating the relationship
Using data from a prominent blockchain research firm, Coinmetrics.io, we explore the relationship between prices, computing power (measured by the hashrate), and network (measured by the number of unique active users). We focus on five major mineable cryptocurrencies (i.e. Bitcoin, Ethereum, Monero, Litecoin, and Dash). In some of our test, we expand our data to include an additional 33 cryptocurrencies. Our sample period spans from 7 August 2015 to 25 January 2019.
We set the stage for our formal econometric analysis by providing some graphical evidence. For example, in Figure 1, we see a close mapping of the prices of Bitcoin and Ethereum with their respective computing power values. Moreover, in Figure 2, we observe an even stronger mapping of the prices of Bitcoin and Ethereum with their respective network values. Interestingly, these graphs also show that when prices significantly exceed the trend in computing power or network (as was the case in late 2017), prices eventually fall and trace the trend in fundamentals. The convergence of prices to the trend with fundamentals suggests that fundamentals are the ultimate, long-run determinants of prices.
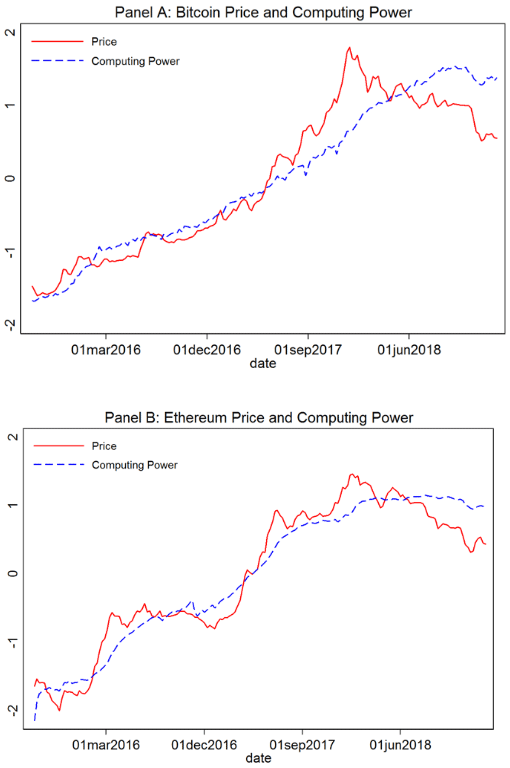
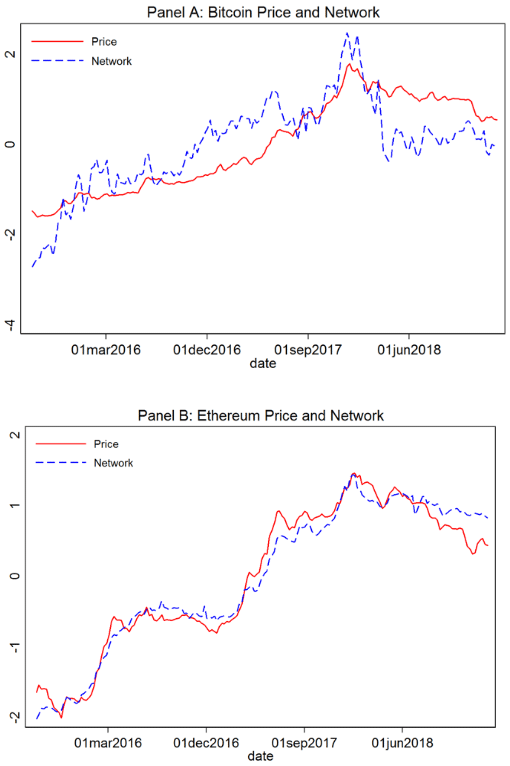
Formal investigation and robustness
We supplement the graphical evidence with a formal estimation that takes into account that prices, computing power, and network, are endogenous variables that are jointly determined at the equilibrium. As such, the graphical evidence might portrait a deceivingly strong positive relationship among them. To resolve this empirical hurdle, we follow Lettau and Ludvigson (2001) and Lustig and Van Nieuwerburgh (2005) and use the dynamic ordinary least squares (DOLS) of Stock and Watson (1993). Our estimation shows that, on average, the prices of five major mineable cryptocurrencies – namely, Bitcoin, Ethereum, Monero, Litecoin, and Dash – depend on their computing power and network. Consistent with existing empirical work (e.g. Corbet et al. 2018, Li et al. 2018, Makarov and Schoar 2018), we also find that there are some periods when prices deviate from the long-run trend with fundamentals.
We further explore the significance of cryptocurrency fundamentals at the aggregate market level using traditional asset pricing tests. For this analysis, we construct risk factors that are based on aggregate values of computing power and network. We denote the aggregate computing power factor with ACP and aggregate network factor with ANET. The innovation in constructing these factors is that we express them in cryptocurrency return units following the factor mimicking portfolio approach (Knez et al. 1994, Lamont 2001, Vassalou 2003).
We also consider two additional factors that account for the finding that in some periods prices drift away from fundamentals. The first factor is the return of Bitcoin. We argue that Bitcoin, being the largest and most traded cryptocurrency, is the most perceptible to sentiment and speculative trading by investors. Thus, as a factor, it can capture periods when trading forces unrelated to fundamentals are the strongest. The second factor is a cryptocurrency price momentum factor that we construct following the seminal work of Jegadeesh and Titman (1993). Momentum effects have been linked to investor psychology (e.g. Barberis et al. 1998).
We test the ability of our factors to price cryptocurrency returns following the asset pricing literature. Asset pricing theory (Cochrane, 2005, 2011) suggests that if the aggregate computing power and network factors are meaningful risk factors for cryptocurrencies, then they should earn positive risk premia. Consistent with this hypothesis, we find that the fundamentals-based ACP and ANET factors have a positive risk premium over the return of the 30-day Treasury bill. In particular, the average weekly returns of the ACP and ANET factors are 1.95% and 1.63%, respectively. We also find that all cryptocurrency factors have higher average returns and standard deviations than the US equity market. However, their Sharpe ratios are comparable to that of the US stock market. For example, the Sharpe ratio of the ACP factor is 14.9% while that of the US equity market is 11%. Overall, the cryptocurrency factors reflect the high reward-high risk trade-off of this market.
More importantly, we find that the ACP and ANET factors can price cryptocurrency returns. In particular, all of our five cryptocurrencies have positive and significant exposures to the fundamental factors. The explanatory power of ACP and ANET is over and above any effects related to the Bitcoin and momentum factors. This finding is non-trivial given that the five cryptocurrencies in our sample have consistently accounted for between 80% to 95% of the total cryptocurrency market capitalisation. Furthermore, we extend our factor analysis to an out-of-sample set of 33 cryptocurrencies obtained from the Bittrex exchange. We find that the aggregate computing power and network factors also price cryptocurrencies in this large out-of-sample data set.
Conclusions
Overall, we offer two sets of novel findings. First, we show that, in the long run, the prices of five major mineable cryptocurrencies depend on their computing power (i.e. hashrate) and network (i.e. number of users). Second, we construct risk factors related to the aggregate values of computing power and aggregate network and show that they can price the returns of a broad set of cryptocurrencies. Our findings have several implications for the debate on cryptocurrencies. To begin with, we show that the prices of major cryptocurrencies over the long term are grounded in reality since they are based on fundamentals. It is possible that other important factors, like regulatory supervision and political risk, might also become important as the cryptocurrency market matures. Regardless, our study is a small step to better understanding the determinants of cryptocurrency prices.
This article first appeared on www.VoxEU.org on August 24, 2019. Reproduced with permission.